What is Medical AI Good For?
by Michael W. Raphael (@MWRaphael), City University of New York · Published · Updated
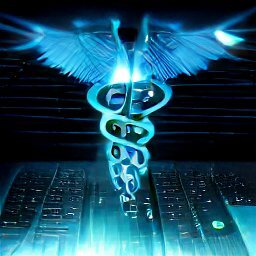
The use of artificial intelligence has grown rapidly, especially in the field of medicine, with the promise of offering advances ranging from more efficient diagnoses to safer treatments. Yet, this promise overlooks the fact that artificial intelligence still faces some pretty serious limitations, you know, the kind of limitations that prevent the machine from operating like we see in television and movies. Simply stated, artificial intelligence is still not yet that intelligent.
Yes, artificial intelligence can do well at particular tasks, such as calculating the best move in a game of chess, generating an image based on a textual description, or seemingly telling jokes as a virtual assistant. But, such an artificial intelligence does not play chess, create the meaning of art, or create improvisational comedy. In the first, second, and third cases, all it does is compute. When we see articles describing some new innovation made possible by artificial intelligence, all the artificial intelligence has done is compute at a scale that is not possible for human beings. Surely, if the machine can calculate at a superhuman capacity, then isn’t it superhuman?
Naturally, the answer is: it depends. If all we do is take the model of a human being to be a calculator, then sure. However, if we take the model of a human being to be a participant in their own reality, then the machine is quite dumb. In other words, as social creatures, humans adapt intelligently not only because we figured out the design of the world around us, but also because we communicate in a way that thoroughly shapes the meaning of that design. That is to say that the reason artificial intelligence remains dumb is that it lacks the intelligibility concerning its means of adaptation in its mode of problem-solving.
Now, I have just introduced three technical terms: “intelligibility,” “means of adaptation,” and “mode of problem-solving.” These technical terms are necessary because the problem underlying the question of intelligence is a question of verifying some sort of depth behind appearances. Accordingly, this transforms the question of intelligence into a question of intelligibility. By “intelligibility,” I am referring to the capacity to make sense out of what we see. In that way, this involves a capacity for visualization and the dynamic changes in the relationship between “looking” and “seeing” in the course of problem-solving. By “means of adaptation,” I am referring to the response to what we see in the course of problem-solving. This is to describe how the problem-solver grasps (a) the organization of conditions and constraints for searching and finding a solution, (b) the constitution and organization of information within the problem-solving process, and (c) the concrete level of abstraction that determines the description, and therefore the selection of the immediate task to be done. By the “mode of problem-solving,” I am referring to the undertaking of implementing the solution once a solution is found. This is to describe the effect and consequences of externalizing the calculations that produced the solution, especially the impact of communication on the capacity to put the externalized solution into practice. These three technical terms thus highlight three points of focus. Intelligibility shows what the problem-solver makes of the problem-situation; the means of adaptation shows what the problem-solver does in response to the problem-situation; and the mode of problem-solving reveals how the solution, once found, either halts problem-solving or continues to require problem-solving past the point of solution. In other words, these technical terms provide us three basic points to evaluate what artificial intelligence does and does not do in the course of problem-solving and in the course of communicating its solutions – at a very general level of abstraction.
Before turning to the case of artificial intelligence in medicine, it is useful to describe one general social situation that is analogous to doctor-patient relationships in a way that highlights these three technical points: playing chess. The social situation of playing chess is quite different from how chess is conceived of within game theory. In game theory, chess is treated as a perfect information game. This means that since both players can see all the pieces, it is assumed that a player has instantaneous knowledge of the utility functions of the pieces, knowledge that allows an optimal move to be selected. In playing chess, players are socially situated at multiple levels, namely at the level of bounded rationality and at the level of skill. The fact that players are socially situated in their bounded rationality means that the size and scope of what players can visualize in memory has a significant bearing on their intelligible sense of what is going on. The fact that players are socially situated in their degree of skill means that the degree of skill a player has is going to affect their imaginative potential for grasping what is going on as well as the foresight of possible futures. In other words, game theory describes chess as a maze designed in a vacuum of discourse: positional imagination allows for the description and analysis of the problem-situation in which all that is left is the computation of the solution. When playing chess, unlike machines, we are not limited to this maze; as humans, we have a choice to merely apply procedural rationality (to model the machine) or to utilize situational rationality (to model human participation in human behavior). If we choose procedural rationality, we select a means of adaptation that reveals reason to be an instrument of design. If we choose situational rationality, we select a means of adaptation in which participation in discourse guides the instrumentality of reason in its mode of problem-solving. This is to understand the significance of how playing is socially situated: to grasp how the presence of an audience has a particularly strong bearing on the style of play and the meaning given to what is visualized in live, fateful, discursive conditions. Situational rationality thus reveals how moves in the maze are consequential and non-recursive. This provides a sharp contrast to the moves made while studying and developing one’s positional imagination, moves that are experimentally subject to optimization through experience and trial-and-error. To draw on Erving Goffman’s study of interaction rituals and frame analysis, the basic point is that there is a basic human predicament of balancing the “substance” and “ceremony” of what is going on in practical ritualistic activity: the selection of a focal point that shapes whatever comes next.
The reader is now rightfully wondering: “what does all this explanation of chess have to do with the instrumentality of artificial intelligence in medicine?” The analogy is actually analytically closer than one might initially think. It was Foucault (in The Birth of the Clinic) who popularized the description of the “clinical gaze” in which “the formation of the clinical method was bound up with the emergence of the doctor’s gaze into the field of signs and symptoms.” Just as a chess player is caught up within the ongoing course of activity of play, so too is the doctor. Accordingly, the doctor faces the exact same diagnostic predicament: “Do I practice medicine according to procedural rationality by adhering to medical guidelines?” or, “Do I practice medicine according to situational rationality in which medical guidelines have to be adapted to the patient’s situation?” The first is analogous to treating chess as game theory in which all a computer has to do is compute; the second is analogous to playing chess. The same limitations apply in both cases.
I have presented the argument this way because it demonstrates the crucial differences between machine problem-solving and human problem-solving that are prevalent even before we entertain the many substantive issues inherent in medical encounters themselves, such as the mistaken assumption about the intelligibility of medical information where guidelines often rely on inconclusive, inscrutable, or misguided evidence (with its potential for unfair discriminatory outcomes and liabilities). In effect, before undertaking the important work of evaluating the consequences of technology for the medical encounter, especially in the arena of diagnostic error, we have to understand the boundary that technology cannot overcome, which is the human using it. Therefore, I argue that the potential of artificial intelligence in medicine lies in its potential to improve situational rationality, a rationality that requires artificial intelligibility. Without artificial intelligibility, the ethical problems are obvious: a machine would be making decisions on a patient’s case without any discursive visualization of the symptoms and signs of the patient’s case – decisions made on the basis of technical indicators in a vacuum of discourse. To be sure, the flutter of highly stressful diagnostic environments does not guarantee that doctors are necessarily any better than machines in engaging in the highly discursive and situational character of medical information, but, as human beings, at least the have the capacity to do so. As things stand, artificial intelligence does not and thus it is not an innovation to improve patient-centered care; rather, it stands merely as a distraction from the boundedness of our rationality in which doctors themselves are still coming to terms with the difficulty of teaching and learning the situational rationality of diagnosis. To this end, I have prepared Figure 1 that outlines a more elaborate heuristic for evaluating diagnostic performance in medical encounters. It should be read along the side of the article, “Artificial Intelligence and the Situational Rationality of Diagnosis: Human Problem-Solving and the Artifacts of Health and Medicine,” now available at Sociology Compass.
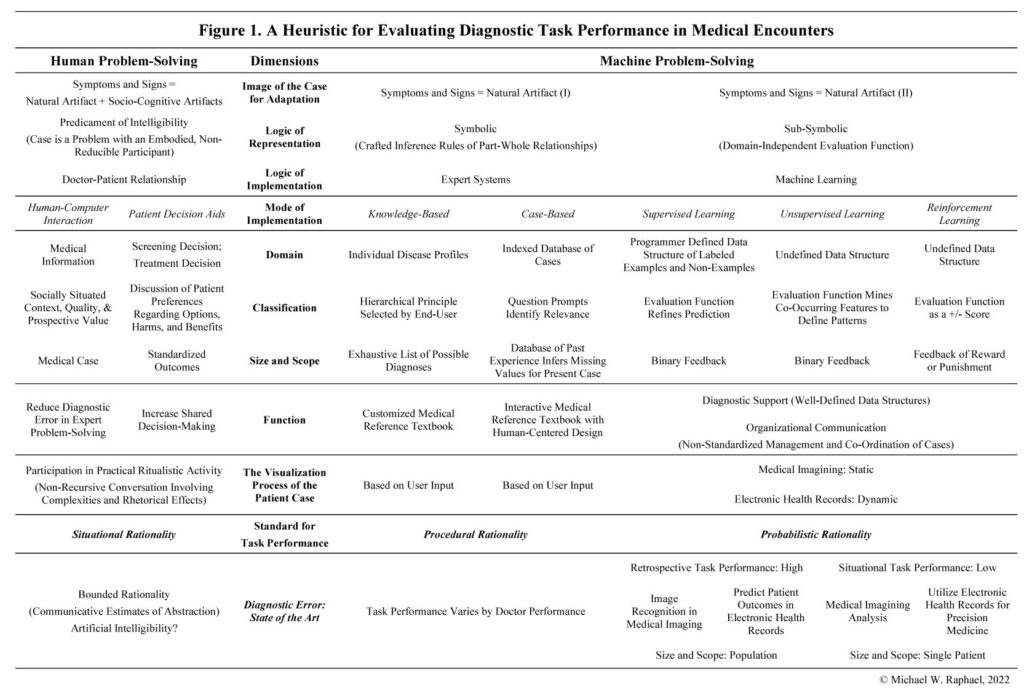
For those willing to entertain a slightly more academic statement about this conclusion, I will end by restating the theoretical point: I am highlighting the contribution of cognitive sociology to the understanding of medical encounters. In other words, diagnostic problem-solving adapts to the unavoidable ambiguities in determining the proper level of abstraction. For the problem-solver, the ambiguities of abstracting present predicaments that require decisions in the course of problem-solving: data can be interpreted (as information) in a number of different ways, and the problem-solver has to decide which sets of constraints to focus on in order to determine what level of abstraction is appropriate, and an adaptation is required to a level of abstraction that is already given in one way or another. The problem-solver’s adaptation to abstraction, then, implies either an adoption a principle of least effort (efficiency) or a principle of most effort (comprehensiveness) in selecting a means of adaptation. In adopting a principle of least effort, the human problem-solver treats the calculation of the solution as constituted in part by heuristics, a response that treats abstraction as essentially reductive in order to grasp the specific components of design, that is, to arrive at a model (the implications of which are intelligible as a matter of practice). In adopting a principle of most effort, the human problem-solver understands several problems must be solved at once – where the calculation of the solution needs to take the limitations of heuristics into account, which is a response of most effort that treats abstraction as essential in determining the intelligibility of whatever is being considered, that is, in the midst of a discourse. It is in this respect that cognitive sociology’s main interest begins wherever design is understood to “end,” for it is at that point of ostensible certainty that problem-solving is understood as a course of activity and not a specific act (an event). This formulation of artificiality in cognitive sociology stands in contrast with philosophical debates in sociological studies of science and technology where artificiality (a possible conclusion) has been developed as a theoretical concept with its own politics regarding the complexity of the issues it discusses, especially the epistemological boundary problem between “the natural” and “the artificial” and the question of “artificial human nature.” In sum, the concern is that artificial intelligence must take into account the fact that human problem-solvers are involved in a problem-solving process, a process in which task performance is thoroughly socially situated in a way that requires artificial intelligibility – and not merely artificial intelligence.
For more information and references, please check out the Open Access publication at Sociology Compass:
Raphael, M. W. (2022). Artificial intelligence and the situational rationality of diagnosis: Human problem-solving and the artifacts of health and medicine. Sociology Compass. https://doi/10.1111/soc4.13047